Surface incoming shortwave (solar) radiation data are an important component ofmany scientific analyses, but direct measurements are not commonly available.Estimates can be obtained from gridded meteorological analysis or reanalysissystems, such as the Global Data Assimilation Systems (GDAS) and Modern EraRetrospective Reanalysis System (MERRA-2), or calculated using empirical modelsdependent on meteorological variables such as air temperature. The purpose ofthis analysis was to compare multiple methods for estimating daily shortwaveradiation in a tropical highland environment in Ethiopia. Direct solar radiationoutputs of GDAS and MERRA-2, topographically corrected outputs of the twoanalysis systems, and empirically estimated solar radiation values calculatedwith the systems’ air temperature data were compared to see which produced themost reliable radiation values. GDAS appeared to underestimate the seasonalvariability, resulting in low correlation (R 2) with insitu data and large mean bias error (MBE).
In comparison, MERRA-2did not underestimate variability, but produced larger bias than the empiricalmodel estimates. There was an improvement in correlation and reduction in MBEwhen using the GDAS air temperature predictions in the empirical model, but theopposite was true for MERRA-2.
The earth’s surface emits a broad spectrum of heat energy upwards as infrared. Within 100 meters, all of the energy in certain frequency bands has been absorbed by CO2 molecules in the air. Those molecules both transfer that energy to adjacent air. In the present study, daily downwelling shortwave (QS) and longwave radiation (QL) data from one satellite and two hybrid products have been.
The empirical model using station air temperaturedata ( stationT) produced the highest correlation across allfour stations, with best performance at the lower elevation sites. The directshortwave radiation outputs of MERRA-2 produced comparable correlation values,with larger R 2 at stations at higher elevation. Topography possiblyinfluenced these results, as MERRA-2 performed comparably tostationT at the stations in moderate terrain, but not insteeper terrain. This work can serve as a starting point for analyses in othertropical highland regions, where continuous in situ solar radiation data arerarely available. 1.1 History & importance of solar radiation dataData on incoming solar radiation at the surface are required for manyapplications, including surface energy balance analyses, generation ofevaporation and transpiration estimates, and site selection for solar energyproduction. However, direct measurements of incoming solar radiation at thesurface are rarely available, so estimates are frequently employed.
Many empirical modelshave been developed to produce shortwave radiation estimates. In someenvironments, relatively simple day of year models provide adequate performance, , using only astronomicaland static geographical (latitude, longitude, topography) inputs. For otherenvironments and applications, however, it is necessary to integrate some kindof meteorological predictors to the model, in the form of sunshine-hour or cloudcover data or as direct meteorological inputs of temperature, precipitation,humidity, and/or other relevant atmospheric conditions ,.The number of sunshine hours and cloud cover are intuitive inputs for a surfacedownwelling shortwave radiation estimate, but they are difficult to measure andare not commonly recorded at most weather stations. Since daily minimum and maximum airtemperature are almost always recorded by professional grade weather stations,many empirical models have been developed to estimate solar radiation as afunction of air temperature ,.Temperature-based models work on the assumption that maximum temperature willdecrease with reduced atmospheric transmissivity, and minimum air temperaturewill increase due to increased cloud cover. Clear skies, in contrast, will resultin higher maximum temperatures due to higher shortwave radiation, and minimumair temperatures will decrease due to lower atmospheric emissivity. Theperformance of different temperature-based estimates can vary quite widely indifferent environments.
Even in cases where the estimates provided by differentmethods are relatively similar, even moderate differences in performance canhave a significant impact when the radiation estimates are used as an input to acrop model.Radiation estimates can also be obtained from surface meteorology analysissystems such as Global Data Assimilation System (GDAS) or Modern-EraRetrospective Analysis for Research and Applications (MERRA-2) ,. These systems assimilate a diversesuite of surface and remotely-sensed observations to advanced meteorologicalmodels, yielding gridded estimates of surface meteorology, including incomingsolar radiation, that are continuous in space and time.
The systems vary in thedetails of the atmospheric model used, the choice of assimilated observationsand the assimilation algorithm, and the number of processes accounted for in thesimulation system. In all reanalysis systems, however, surface incoming solarradiation is calculated as an outcome of atmospheric radiative transferprocesses rather than estimated as a function of other near-surface variables.Previous work has shown that reanalyses products contain considerableuncertainty in the estimate of solar radiation at daily timescales , though no analysis hasbeen performed in tropical highland regions.A third class of radiation estimates are those derived from satelliteobservations (e.g., ). These methods make use of satellite observed upwelling shortwaveradiation at the satellite, which, in combination with independent informationon surface albedo, cloud properties, and atmospheric radiative transfer, can beused to estimate downwelling radiation at the surface. These methods areincreasingly applied for solar radiation monitoring and have been leveraged forshort-term radiation forecasts. These methods are not included in this study, asour focus is on comparing advanced reanalyses to station-based empirical methodsin a highly cloud affected region, with an eye to applications in seasonalforecasts and climate projections. 1.2 Region of study: Northern Ethiopian HighlandsThe Ethiopian Highlands are a densely populated, mostly rural region, dominatedby smallholder subsistence agriculture.
Local climate variability is extreme:steep, dissected topography and a dominance of convective precipitationprocesses mean that temperature, rainfall, and other meteorological conditionscan vary significantly over distances of just a few kilometers. Meteorologicalmonitoring under such conditions is a considerable challenge, and the importanceof this challenge is underscored by the climate vulnerability of the localfarming population ,.In this context, the importance of rainfall monitoring – and, to some extent, temperaturemonitoring , , have been consideredat some length.
Here, we consider the problem of estimating local incomingshortwave (solar) radiation. This variable is relevant for multiple applicationsin climate, agriculture, and energy analysis, and it stands out as aparticularly important variable in that it is a required input to manyprocess-based crop models. Publicly available in situmeasurements of incoming shortwave radiation are almost completely absent in theEthiopian Highlands, and steep topography makes it difficult to extrapolate fromany single measurement site with confidence.The objective of this study was thus to determine the best way to estimatedaily-received solar radiation when there are no in situmeasurements available. The direct output of meteorological analysis systems,topographically corrected meteorological analysis outputs, and empiricallyestimated solar radiation values calculated with meteorological analysistemperatures were compared to see which produced the most reliable results. 2.1 StationsWeather station air temperature and solar radiation data from four sites in theEthiopian Highlands were used in this study.
The Kurar station is located in thesteepest terrain and the lowest elevation of the four sites, with the hottestrecorded temperatures. The Debre Markos and Yejubie stations are located in therolling hills, while the Enebi station is located on a flat plain. All data werecollected using Davis Instruments Vantage Pro2 weather stations installed at 2 mheight on tripods sited in the middle of open fields. A fifth station installednear the top of the mountain failed during data collection and is not includedin this study. Site nameLatitudeLongitudeElevation (m)Time period of recorded dataKurar10°06’00”38°5/2/2014–11/3/2014, 1/1/2015–1/31/2015Yejubie10°12’00”37°5/2/2014–,1/27/2015–4/8/2015,5/16/2015-6/20/2015Enebi10°14’17”38°5/2/2014–7/14/2014, 8/5/2014–9/14/2014,11/3/2014–1/28/2015,4/2/2015–6/29/2015,7/17/2015–8/13/2015,1/14/2016–4/29/2016Debre Markos10°18’00”37°6/6/2014–12/8/2014,1/1/2015–5/29/2015,8/29/2015–, 3/6/2016–6/21/2016.
Model selectionAlmorox tested fifteendifferent solar radiation empirical models, categorized based on the primarymeteorological variable used. The models were tested using weather station datafrom Spain, and the coefficient of determination (R 2), root meansquare error (RMSE), and mean bias error (MBE) was compared to evaluate whichtype of model made the most accurate solar radiation estimates. Mechwarrior online discord games.
Almorox has alsotested air temperature empirical models in other locations in Spain , Venezuela , and Argentina. In this analysis in theEthiopian Highlands, four of the temperature models discussed in the literaturestudy were tested: Hargreaves & Samani , Bristow & Campbell , Donatelli &Campbell , and theGoodin model.
Whileit has been acknowledged that temperature-based models produce less accurateestimates than cloud or sunshine-based models , air temperature is more commonlymeasured at weather stations. The results showed that out of all thetemperature-based models tested with the data from Spain, the Bristow &Campbell model produced the most accurate estimates. This influenced the decision to testthe Bristow & Campbell model and its other forms (Donatelli &Campbell and Goodin models) with theEthiopia air temperature data. The Hargreaves & Samani model was also tested due toits simplicity. All of the methods tested in this study are process-basedstructured equations—i.e., the form of the relationship is fixed based onhypothesized and/or observed relationships between the meteorological predictorsand the response variable.
We note that in recent years a number ofinvestigators have applied machine learning and data mining approaches to theproblem of estimating shortwave radiation, and these new methods have shown goodperformance in several cases –. Wechoose to focus on the structured equations since they are established andrelatively easily applied methods, and they provide a useful point of comparisonto the globally available reanalysis products that we also evaluate. (7)The change in temperature is calculated as the differencebetween a day’s maximum temperature and the minimum temperature of the sameday (generally early morning, local time) and the following day. The generalinterpretation is that the A parameter represents the average clear-skytransmissivity, and parameters B and C are dependent on the recorded airtemperature.
Theaccuracy and simplicity of this model has made it great source of solarradiation estimates at sites that have no data available. 2.4 Analysis-based radiation estimatesAnalysis datasets—GDAS and MERRA-2—were applied to generate incoming solarradiation estimates in three ways. First, the standard incoming surface solarradiation variable from each analysis product was extracted for each study site.Second, the analysis was downscaled from each product’s native resolution (0.25°for GDAS during the period of analysis, and 0.5° x°0.625 for MERRA-2) to 5 kmhorizontal resolution using a slope-aspect correction adopted from Dingman to account forthe influence of sun angle on incoming direct radiation, and these slope-aspectcorrected radiation values were extracted for each site. Third, to mimic thestation-based empirical method, a hybrid approach was used in which the GDAS andMERRA-2 2 m air temperature fields were used to estimate surface radiation withthe Goodin empirical model. The purpose of these comparisons was to assess arange of ways in which analysis data can be used to generate estimates ofR S.
Hargreaves & SamaniABCKurar0.17Yejubie0.15Enebi0.16Debre Markos0.17Average0.16Bristow & CampbellABCKurar0.700.021.86Yejubie0.630.081.25Enebi1.040.041.16Debre Markos1.900.080.59Average1.070.061.21Donatelli & CampbellABCKurar0.700.4233.30Yejubie0.570.3013.64Enebi0.690.3327.95Debre Markos0.630.3916.07Average0.650.3622.74GoodinABCKurar0.711.011.74Yejubie0.612.601.33Enebi0.891.911.19Debre Markos0.795.990.84Average0.752.881.27. MethodStation nameMaximum (W/m 2)Minimum (W/m 2)Mean (W/m 2)SD (W/m 2)rRMSE (W/m 2)MBE(W/m 2)Hargreaves & SamaniKurar271.8125.0221.7.1-17.5Yejubie282.5117.4224.8.619.7Enebi294.8100.0224.6.6-9.2D. Markos293.9113.6217.1.0-1.5Average285.7114.0222.0.3-2.1Bristow & CampbellKurar366.0108.3294.8.055.5Yejubie388.995.2297.42.292.3Enebi396.698.7299.4.665.6D. Markos392.9120.0288.1.069.5Average386.1105.6294.9.770.8Donatelli & CampbellKurar275.253.4226.7.1-12.6Yejubie275.241.1222.3.817.2Enebi277.944.1222.3.6-11.5D. The Bristow & Campbell model produced the largest RMSE, mean bias error, and standarddeviation out of all the temperature-based models tested. The differences incorrelation between the four tested models were small.
However, the Goodin modelstatistical results fell more in the middle, as it produced maximum, minimum,standard deviation, RMSE and bias error values that were not extremely large orsmall compared to the other models. For this reason, and due to its demonstratedpotential at non-instrumented sites , the Goodin model was selected togenerate the empirical radiation estimates. All of the following empiricalresults discussed refer to estimates generated using the Goodin model.
3.2 Temperature comparisonThe empirical solar radiation estimates were calculated using daily maximum andminimum air temperature. Therefore, we examined the temperature station data andcompared it to GDAS and MERRA-2 records. The GDAS and MERRA-2 temperature valuesdid not change when applying the slope-aspect correction. The maximum, minimum,mean, and standard deviation are shown in, while the correlation, RMSE, and MBEof each set of temperature estimates for each station are reported in. GDAS–Maximum TemperatureKurarYejubieEnebiD. MarkosAverager0.780.800.820.880.82RMSE (°C)1.901.91.61.61.74MBE (°C)0.130.50.2-2.1-0.31Standard deviation (°C)2.52.62.22.62.5MERRA-2 –Maximum TemperatureKurarYejubieEnebiD.
MarkosAverager0.810.820.870.860.84RMSE (°C)2.663.03.22.92.94MBE (°C)-1.912.22.72.31.31Standard deviation (°C)3.13.73.53.53.4GDAS–Minimum TemperatureKurarYejubieEnebiD. MarkosAverager0.680.510.690.720.65RMSE (°C)2.902.02.01.32.04MBE (°C)-2.311.20.9-0.1-0.10Standard deviation (°C)2.41.92.01.82.0MERRA-2 –Minimum TemperatureKurarYejubieEnebiD. MarkosAverager0.600.540.810.740.67RMSE (°C)1.823.53.83.03.03MBE (°C)-0.983.23.52.72.11Standard deviation (°C)1.81.52.22.11.9.
Overall MERRA-2 tends to produce warmer maximum and minimum temperature valuesthan GDAS and the station recorded data. This is also reflected in the meanbias, as GDAS has a smaller, negative average bias while MERRA-2 has a larger,positive (warm) bias. MERRA-2 has larger RMSE than GDAS for both maximum and minimumtemperatures. MERRA-2 is more variable than GDAS for the maximum temperature,but the difference in standard deviation is not as large for the minimumtemperature. In terms of correlation, MERRA-2 and GDAS produce similar values.The only large difference in correlation values is for the minimum temperatureat Enebi.
3.3 Solar radiation estimatesDue to limitations in field access to download data, particularly outside of themajor cropping seasons, the time records at the four weather stations arediscontinuous. Nevertheless, some seasonal and sub-seasonal patterns are evidentin the solar radiation record. In most but not all cases there is a dip in solar radiationduring the wet season (June-September), though the cloudiness effect is offsetby the fact that the stations are in the Northern Hemisphere and so get a slightincrease in potential incoming solar radiation in boreal summer. To first order,this temporal variability is captured by empirical methods and appears to becaptured by MERRA-2. GDAS tends to underestimate seasonal variability, thoughhybrid estimates that use GDAS temperature and empirical solar radiationalgorithms do capture seasonal effects. Solar radiation estimates and in situ data for a.) Kurar, b.) Yejubie,c.) Enebi, and d.) Debre Markos stations.We also see that GDAS has the highestR S estimates , and that theseestimates are biased high relative to station observations. MERRA-2R S also tends to exceedobserved values, though not as severely as GDAS.
Empirical estimates based onin situ temperature measurements perform much better interms of bias but underestimate the range of observed values. In comparison,MERRA-2 does not underestimate the range of values, but has larger bias.
Thehybrid estimates that utilize the analysis system temperatures (both GDAS andMERRA-2) have a reduction in range values compared to the direct radiationoutputs. 3.4 Evaluation of radiation estimatesFor all stations, the empirical model based on in situ stationtemperature measurements ( stationT) provided the highestcorrelation with observed R S (, blue bars in ). For Kurar, which islocated on steep topography that poses a challenge for relatively coarseanalysis products, this advantage was dramatic. StationT wasbetter than almost all other methods by a statistically significant margin (p.
The solar radiation estimates taken from GDAS had the largest mean bias erroracross all stations.There was also a decrease in MBE when using the temperature-based estimatesinstead of the system radiation outputs (for both GDAS and MERRA-2). Thedecrease in bias error was more noticeable for GDAS, which complements theobserved improvement in correlation and RMSE when using GDAS temperature-basedestimates instead of the direct GDAS radiation values. The MERRA-2temperature-based estimates produced a negative bias in Kurar, possibly due tothe warm temperature bias.Similar to the mean bias results, GDAS had the largest root mean square errorvalues across all stations. For both GDAS and MERRA-2, there was a decrease in RMSE whenusing the temperature-based estimates instead of the direct radiation outputs.Furthermore, the GDAS and MERRA-2 temperature-based estimates( GDAS/MERRA-2 rawT & slopeT) producedsimilar RMSE values, and GDAS rawT/slopeT had RMSE valuesslightly closer to the empirical model in situtemperature-based estimates ( stationT). It is understandablethat the two systems’ temperature-based estimates produced similar RMSE valuessince they performed comparably for the air temperature predictions ( & ).
The proximity inthe RMSE values for GDAS and stationT could be explained by the lowertemperature bias for GDAS than MERRA-2. Similar to the correlation, theimproved performance of stationT estimates was most noticeableat Kurar, with it having the smallest RMSE. 3.4.1 Influence of parameters on estimatesThe results reported above were generated using the average of thecoefficients at all sites for the temperature-based estimates of solarradiation (see ). To test sensitivity to local coefficients, we repeated thecalculations using the site-specific coefficients.The ‘site-based’ results (green bars in ) showed that there are very smalldecreases in correlation when using the average of the site-basedcoefficients for all locations. However, there are more noticeable changesin mean bias when using the site-based coefficients. All of the empiricalmodel estimates have an improvement in MBE at the Yejubie and Enebi stationswhen using the individual site parameters.
At the Kurar station, there is areduction in mean bias error for the stationT and GDAStemperature-based estimates, but an increase in MBE for MERRA-2temperature-based estimate. 4.1 Temperature comparisonThe GDAS maximum and minimum temperature predictions typically had a smallerstandard deviation than MERRA-2 temperature values. Since MERRA-2 had a largervariation in temperature estimates, one thought that could be considered is thatMERRA-2 could be more capable of capturing Ethiopia’s complex climate andtemperature range. Despite the large standard deviation, however, MERRA-2 tendedto have warmer temperatures than the station data.
Furthermore, GDAS had smallerbias and root mean square error than MERRA-2. Having a larger standard deviationor a reduced bias and error does not seem to have a strong relationship with themodel’s ability to capture temperature variability, as GDAS and MERRA-2performed comparably in this region when comparing correlation values. Despitethe complexity of the region’s climate and topography, both analysis systemscapture the temporal pattern of variability about equally as well.There is a general agreement that these temperature-based empirical models canmake reliable solar radiation estimates ,. However, one factor that should betaken into consideration is that the temperature-based empirical models assumethat air temperature is the dominant factor that influences incoming solarradiation. Otherfactors could muddle the relationship between air temperature and incomingshortwave radiation, such as topography, wind speed, water vapor, and weathersystems, and therefore influence the accuracy of temperature-based empiricalmodels at higher elevations. These complicating factors may be particularly relevant in atropical highland region, where convection can be intense and highlylocalized. 4.2 General patterns in solar radiation estimation comparisonThe GDAS estimates had the highest means across all four stations, but smallerstandard deviations, larger RMSE and mean bias, as well as lower correlations tothe in situ data.
The GDAS solar radiation estimates were generally too large,and limited to a higher range of values. The improvements in correlation andreduction in RMSE and MBE when using the temperature-based estimates from GDASinstead of the direct radiation values indicated that if planning to obtainsolar radiation estimates from GDAS, it is better to use the predicted airtemperature in the empirical model than to use the shortwave radiationestimates. MERRA-2 also had large mean values (when compared to the mean of thein situ data), but generally had large standard deviations,which seemed to make the estimates more comparable to station radiation valuesfor this region.When comparing the two best methods (in terms of correlation), MERRA-2 had arange similar to the station data, but also had a large bias.
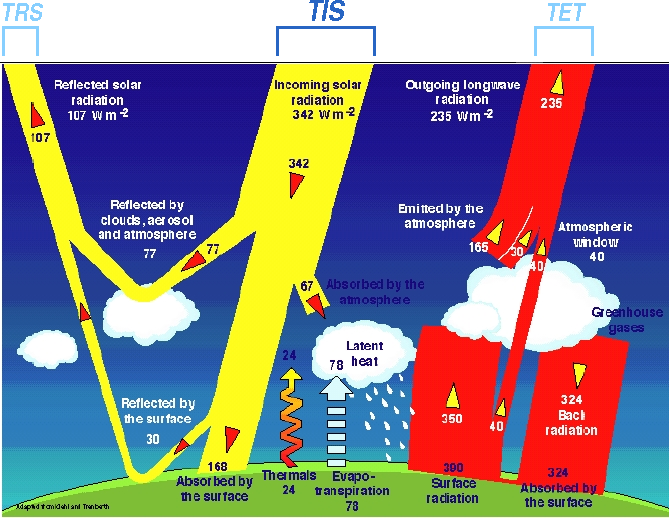
ThestationT estimates had a limited range in radiation values,but a smaller bias. Using the temperature estimates from MERRA-2 (or GDAS) inthe empirical model could be seen as a middle ground, as it reduced the range ofvalues, but it also improved the mean bias.
When just looking at correlationvalues, MERRA-2 performed comparably to stationT at the Enebistation, and also performed well at the Debre Markos and Yejubie stations. Therewas only a drastic difference in correlation between those two sets of estimatesat the Kurar station, which was located in the steepest terrain of the foursites. This could indicate that MERRA-2 performs better in areas of flattertopography. In contrast, the correlation of stationT decreasedat the higher elevation sites. This could suggest that the empirical model doesnot perform well in areas of higher elevation, possibly due to its limited rangeof radiation values. The complex climate and elevation profile in the EthiopianHighlands most likely challenged the ability of reanalysis systems to makeaccurate solar radiation predictions in this region.
4.3 Raw output v. Slope corrected output of GDAS and MERRA-2The differences between the mean and standard deviation of the raw andslope-corrected outputs of GDAS and MERRA-2 were small. The changes incorrelation between the raw output and slope-corrected outputs of the analysissystems were also minimal. There was essentially no change in correlation in theMERRA-2 sets of estimates, while there were very small changes in correlation atthe Kurar and Enebi stations for the GDAS estimates. While there were no largechanges in correlation when comparing the raw output and the slope-correctedoutput of the reanalysis systems, there were slight changes in mean bias error.
At the Kurarand Enebi stations, the raw output of both GDAS and MERRA-2 had slightlyimproved MBE, while at the Yejubie and Debre Markos stations, theslope-corrected output of GDAS and MERRA-2 had improved MBE. A possibleexplanation for this is related to the topography at the site locations. It isreasonable that the raw output of these systems would have improved mean biaserror at the Enebi station since there is no slope in the topography there. TheKurar station is located in the steepest terrain, so it is possible that theslope-corrected output does not capture highly localized topography, resultingin the raw output having better estimates. The Yejubie and Debre Markos stationsare both located in the rolling hills, which have a moderate change intopography and therefore the slope correction is able to provide more accuratesolar radiation estimates. 4.4 Site-based coefficients and leave-out analysisIt is reasonable that there was a slight improvement in correlation at the higherelevation sites (Debre Markos and Enebi) when the Kurar parameters (the lowestelevation site) were not considered in the average. The very small improvementindicated that using the average coefficients did not cause a large decrease inaccuracy of shortwave radiation estimates.
While the changes in correlation whenusing the site-based coefficients were small, there were some notable changes inmean bias error. These changes in mean bias error also occurred when conductingthe ‘no-Kurar’ analysis. The increase in MBE for the MERRA-2 temperatureestimates at the Kurar station (for both the site-based and the ‘no Kurar’tests) supports the idea that MERRA-2 does not perform well in locations ofsteep topography. Though there was an improvement in mean bias error at theDebre Markos station, which is located in the hills region of Ethiopia, possiblysuggesting that MERRA-2 can handle some smaller changes in topography. 5.0 ConclusionsSolar radiation estimates are a critical component of many scientific analyses, butin situ data can be hard to obtain. Empirical temperature-basedmodels provide one way of generating accurate estimates with easily obtainable data.Meteorological analysis systems such as GDAS and MERRA-2, also serve as a source ofsolar radiation values. The goal of this study was to see which source of estimatesprovided the most reliable shortwave radiation values for a tropical highlandregion.StationT produced the highest correlations to insitu data, but the MERRA-2 raw outputs also performed well.
It appearedthat stationT performed best in the areas of lower elevation, whilethe raw output of MERRA-2 had improved solar radiation estimates in areas of higherelevation. It is possible that topography played a role, as MERRA-2 performedcomparably to stationT at the Enebi (flat plain), Debre Markos andYejubie (rolling hills) stations, but not so at the Kurar station (steepestterrain).This study provides a basis for selecting a shortwave radiation estimate whenapplying crop models, studying land-atmosphere interactions, or mapping solar powerpotential in the Ethiopian highlands. The comparisons performed here can also informwork elsewhere in the highland tropics, where consistent in situ solar radiationobservations are rarely available.
Future work could include expanding the testingrange beyond the highlands to other regions of Ethiopia. Since the climate ofEthiopia is complex, future research could also include testing other empiricalmodels that use other weather variables such as rainfall, though this is dependenton the records available at the weather stations.